1 yr
Exploring the Power of Natural Language Processing (NLP) in Data Science Applications. Natural Language Processing (NLP) is a subfield of artificial intelligence that focuses on the interaction between computers and human language. It enables machines to understand, interpret, and generate human language, opening up a wide range of possibilities for data science applications. NLP has gained immense popularity in recent years due to its ability to extract valuable insights from the vast amount of unstructured text data available on the internet and in various organizational databases. In this article, we will explore the power of NLP in data science applications. Text Analysis and Sentiment...
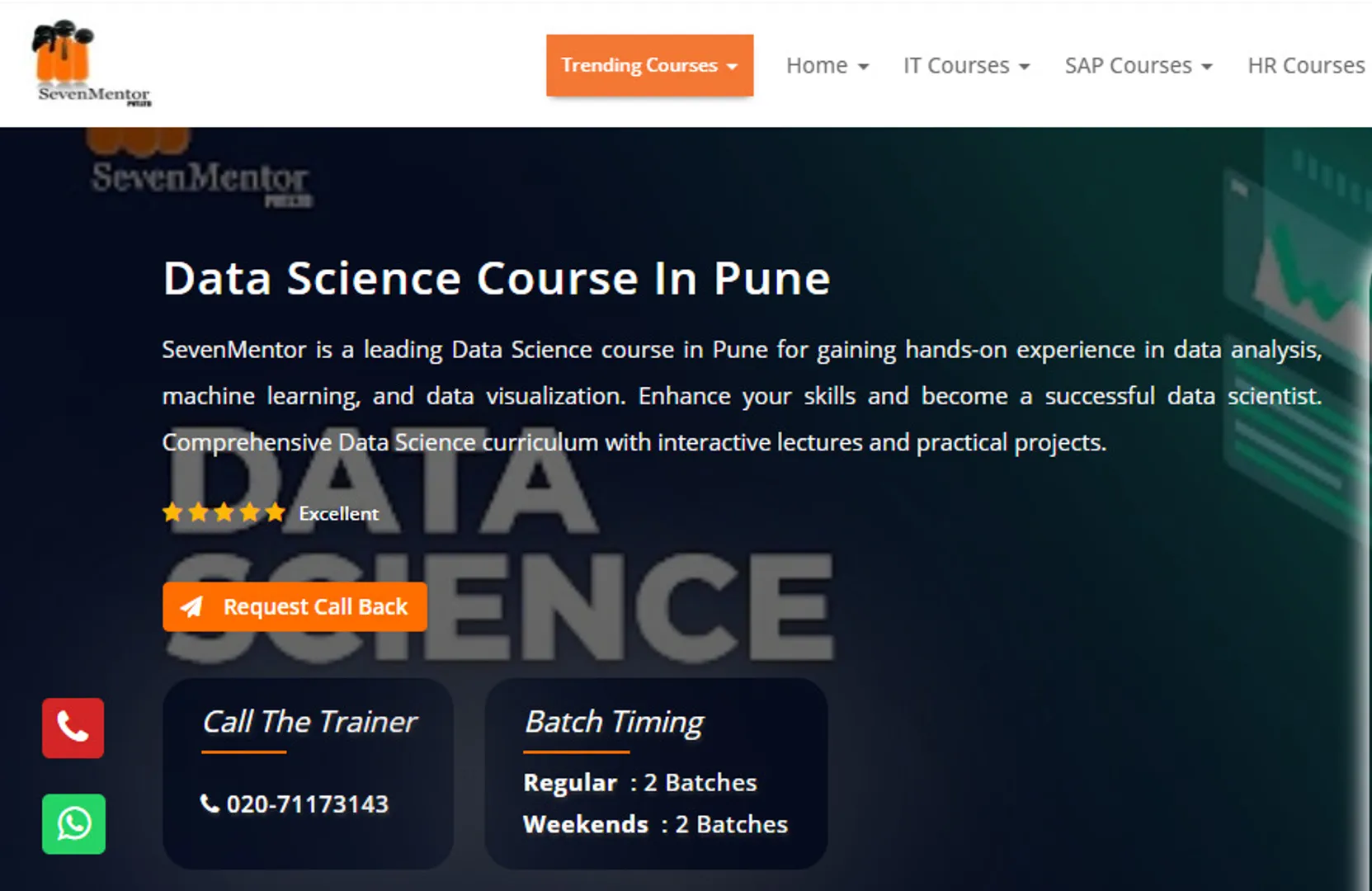